英特爾攜手健培科技加快醫(yī)學(xué)影像人工智能診斷速度
健培科技在醫(yī)療影像AI領(lǐng)域持續(xù)創(chuàng)造出色的成績,除了自身強(qiáng)大的研發(fā)實(shí)力之外,還得益于各大合作單位的通力協(xié)作。
近日,英特爾官網(wǎng)公布了與健培科技進(jìn)行技術(shù)攻關(guān)的成績單,僅基于人工智能平臺(tái)的圖像讀取性能上就提升了8倍。
(圖為英特爾官網(wǎng)全球報(bào)道)
全文翻譯
In China, Artificial Intelligence (AI) has deeply penetrated many industries, including medical care. This is motivated by the fact that 80 percent of medical data in China is imaging, which requires analysis and diagnosis based on the patient scans.
在中國,人工智能已經(jīng)深入到包括醫(yī)療在內(nèi)的許多行業(yè)。醫(yī)療數(shù)據(jù)中,80%都是影像數(shù)據(jù),而影像數(shù)據(jù)需要根據(jù)患者掃描結(jié)果進(jìn)行分析和診斷。
China faces two challenges with image processing: 1) there are not enough practitioners for human review to keep up with the growth of image volume, which currently expands by 30 percent per year; and 2) current computer-aided analysis lacks accuracy; manual review and subjective interpretation is inevitable.
中國在醫(yī)療影像處理方面面臨兩大挑戰(zhàn):1.沒有足夠的從業(yè)人員進(jìn)行人體檢查以滿足圖像量的增長,目前圖像量每年以30%的速度增長;2.目前的計(jì)算機(jī)輔助分析的準(zhǔn)確性尚未獲得驗(yàn)證;人工審查不可避免。
AI in Medical Imaging Science
醫(yī)療影像學(xué)人工智能
The application of AI inferencing in medical imaging is complicated, requiring powerful processing capabilities for such challenges as data diversification, deep analysis, and complicated labeling. Medical image analysis requires support of 3D?or even 4D?deep neural network (DNN) architectures, which rely heavily on platform memory during processing. GPUs are often unable to handle the tremendous number of workloads required to process 3D and 4D image data. Therefore, medical teams may reduce the pixels of 3D image data and split them into multiple small blocks for sequential recognition. The TensorFlow* deep learning framework used to train algorithms on imaging data can benefit from optimizations for Intel? Xeon? Scalable processors.
將人工智能應(yīng)用于醫(yī)療影像推理是非常復(fù)雜的,需要強(qiáng)大的處理能力來應(yīng)對數(shù)據(jù)多樣化、深度學(xué)習(xí)和復(fù)雜標(biāo)記等挑戰(zhàn)。醫(yī)療影像分析需要支持3D甚至4D深度神經(jīng)網(wǎng)絡(luò)(DNN)體系結(jié)構(gòu),在處理過程中嚴(yán)重依賴于平臺(tái)內(nèi)存。GPU通常無法滿足處理3D和4D影像數(shù)據(jù)時(shí)所需的大量工作負(fù)載。因此,醫(yī)療團(tuán)隊(duì)通常采用減少3D影像數(shù)據(jù)的像素,并將其分割成多個(gè)小的影像塊進(jìn)行順序識(shí)別。用于訓(xùn)練成像數(shù)據(jù)算法的TensorFlow*深度學(xué)習(xí)框架可以從英特爾至強(qiáng)可擴(kuò)展處理器的優(yōu)化中獲益。
The Intel Xeon Scalable processor family is well-suited for deep learning applications. It can directly read up to 384 GB of memory to accommodate fast access to imaging data for inferencing. Thus, it can better meet the requirements of AI-based CT image analysis compared to other AI processing technologies.
英特爾可擴(kuò)展處理器系列非常適合深度學(xué)習(xí)應(yīng)用程序。它可以直接讀取高達(dá)384GB的內(nèi)存,以便快速訪問影像數(shù)據(jù)進(jìn)行推理。因此,與其他人工智能處理技術(shù)相比,它能更好地滿足基于人工智能的CT圖像分析的要求。
Intel? Optimization for TensorFlow* leverages the Intel? Math Kernel Library for Deep Neural Networks (Intel? MKL-DNN) to help improve performance on image data processing. Plus, it has customized 5,000 new features to provide better support for medical image analysis issues.
英特爾TensorFlow*優(yōu)化利用英特爾深度神經(jīng)網(wǎng)絡(luò)的核心庫(英特爾MKL-DNN)幫助提高影像數(shù)據(jù)處理性能。此外,它還定制了5000個(gè)新功能,以更好地支持醫(yī)學(xué)圖像分析問題。
Introducing an Image-Reading Robot
介紹一種影像閱片機(jī)器人
JianPei Tech LTD’s image analysis robot is built on advanced AI algorithms and deep learning technologies, enabling highly accurate image recognition. Assisting doctors in locating diseases, analyzing conditions, and guiding operations, the image reading robot is part of a clinical decision-making system and is at the frontier of medical science and medical technology development within the country. With the AI-enabled robot, the time needed to complete radiologist diagnoses have reduced immensely. This application has also substantially increased diagnostic accuracy using Intel optimizations.Such impact can advance precision medicine greatly in China.
在中國,人工智能在醫(yī)療領(lǐng)域的應(yīng)用從手術(shù)機(jī)器人、醫(yī)學(xué)影像診斷到遠(yuǎn)程醫(yī)療等細(xì)分領(lǐng)域經(jīng)歷了從無到有,從小到大的跨越式的發(fā)展。人工智能對醫(yī)療行業(yè)不僅僅是顛覆,更多的是創(chuàng)新。健培科技與英特爾合作實(shí)際上就是對醫(yī)療行業(yè)的一次顛覆性創(chuàng)新。在現(xiàn)實(shí)應(yīng)用中,健培閱片機(jī)器人的診斷速度、準(zhǔn)確率等主要指標(biāo)均處于行業(yè)領(lǐng)先地位。它的出現(xiàn),除了提高醫(yī)生的工作效率外,還將作為輔助診斷,大大提高診斷的效率和準(zhǔn)確率。健培閱片機(jī)器人可以協(xié)助醫(yī)生定位疾病、分析病情、指導(dǎo)手術(shù),是臨床決策系統(tǒng)的一部分,處在國內(nèi)醫(yī)學(xué)科學(xué)和醫(yī)學(xué)技術(shù)發(fā)展的前沿地位。
Accelerating AI-Enabled Imaging Analytics and Diagnoses
加速人工智能的圖像分析和診斷
Intel worked with JianPei Tech to optimize their image- reading robot on Intel Xeon Scalable processors for inferencing of X-ray, CT, MRI, and other medical imaging sources. To accelerate image analysis, JianPei Tech’s plat-form was migrated to Intel? Xeon? Gold 6140 processors and optimized with Intel Optimization for TensorFlow, which includes the Intel MKL-DNN library.
英特爾與健培科技合作,優(yōu)化英特爾至強(qiáng)可擴(kuò)展處理器上的影像閱片機(jī)器人,以推理X射線、CT、MRI和其他醫(yī)學(xué)成像數(shù)據(jù)。為了加快圖像分析,健培的平臺(tái)被移植到英特爾?至強(qiáng)?金 6140處理器,并使用英特爾? TensorFlow優(yōu)化庫(包括英特爾? MKL-DNN庫)進(jìn)行優(yōu)化。
As shown in Figure 1, the collaborative work resulted in achieving an 8X performance improvement on DICOM (Digital Imaging and Communications in Medicine) images (identified as DCM in Figure 1).1 The speedups were a result of using Faster R-CNN* (Region Convolutional Neural Network) instead of UNet* and optimizing the Faster R-CNN algorithms for Intel Xeon Scalable processors.
如圖1所示,協(xié)作工作使DICOM(醫(yī)學(xué)數(shù)字成像和通信)圖像(如圖1所示為DCM)的性能提高了8倍。
英特爾?TensorFlow優(yōu)化推理吞吐量與英特爾?志強(qiáng)?金 6140處理器上的UNET和自定義Faster R-CNN相比
圖1使用優(yōu)化的Faster R-CNN算法推理性能吞吐量
Faster R-CNN has also played a role in segmentation analytics in the medical field. Faster R-CNN is well optimized by Intel Optimization for TensorFlow and Intel MKL-DNN, which results in a performance gain of 6X compared to UNet* (see Figure 2).
Faster R-CNN也在醫(yī)學(xué)領(lǐng)域的細(xì)分分析中發(fā)揮了作用。Faster R-CNN通過英特爾針對TensorFlow和英特爾MKL-DNN的優(yōu)化得到了很好的優(yōu)化,與UNET*相比,其性能提高了6倍(見圖2)。
英特爾?TensorFlow優(yōu)化推理吞吐量與在英特爾?至強(qiáng)?金6140處理器上自定義Faster R-CNN對比
圖2與英特爾?至強(qiáng)?金 6140處理器上的UNET相比,被TensorFlow優(yōu)化的Faster R-CNN推理性能優(yōu)化更快
Optimum memory capacity and image data batch size also enhanced processing performance for faster R-CNN as shown in Figure 3.
最佳的內(nèi)存容量和圖像數(shù)據(jù)批量大小也提高了Faster R-CNN的處理性能,如圖3所示。
英特爾?TensorFlow優(yōu)化推理吞吐量與英特爾?至強(qiáng)?金 6140處理器上不同批量大小的自定義Faster R-CNN相比
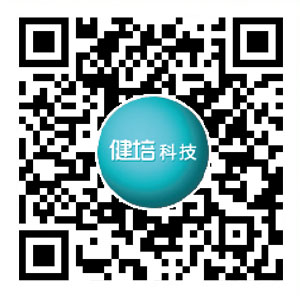
微信掃一掃
關(guān)注該公眾號